Using AIOps for Better Incident Management
Efficiency is key, and as the amount of data, alerts, and incidents keeps increasing, humans alone are not capable of keeping up with the pace. Organizations across the globe are looking towards AIOps and automation to drive towards faster MTTR and less customer impact during incident response.
AIOps can help all teams – from DevOps and distributed service owning teams to ITOps and NOC teams – automate workflows for smarter and more efficient incident management.
In this article, we’ll take a look at how AIOps can improve incident management throughout the incident lifecycle, as well as some of the top AIOps tools available for incident management.
How AIOps is Better for Incident Management
During incident response, it’s common for event data to be sent to a centralized ITOps team or NOC. These first line responders have traditionally employed an eyes-on-glass method to understand the signals coming into their ecosystem. Then, when a relevant signal came past their desk, they’d route it to a response team (catch and dispatch). Now, however, there are too many alerts for humans to keep up and understanding what alerts go where is a challenge.
And, once things are routed to service owning teams, those teams become inundated with alerts themselves. They need to work hard to understand where the problem is coming from, how serious it is, and what needs to be done to resolve it. And a lack of automation means they’re doing this all by hand with toil aplenty.
Analyzing and communicating large amounts of different data points is a large and tedious task for any human. As services and infrastructures become more complex, so too do the data sources. Incident management can quickly become a lot for a single team to handle, so the obvious option was often to simply scale the team. But now, even scaling the team cannot meet the demand. Artificial intelligence can help teams effectively make sense of their data without relying solely on overworked team members.
This is where AIOps truly shines. AIOps stands for Artificial Intelligence for IT Operations. Using data science and artificial intelligence to analyze all of the given data from your IT operations and DevOps tools, AIOps is able to provide response teams with AI-backed insights and intelligence. Here’s how teams can leverage AIOps across the incident lifecycle:
-
- Deduplication and suppression: When an event comes in, that does not automatically mean it needs to be a discrete incident. Sometimes, events actually need to be combined into a single incident. Or, nobody needs to be notified at all. Transient alerts that will resolve themselves within a few minutes can be ignored.
- Noise reduction: Sometimes, alerts can be grouped together within the same incident. While you can do this manually, it’s easier to leverage ML to group intelligently based on previous incident data. Or, you can create your own rules based on content or time to group alerts as you see fit to reduce your incident numbers.
- Event routing, enrichment, and automation: Event data isn’t always the most thorough. Enriching the event means that when the response team gets it, it’s the most helpful it can be. And creating automation that routes and enriches the alert based on criteria and complex logic means that the right team gets an event with the right information right away, without needing manual human input.
- Machine response: Even after an event is routed to its intended destination, there may not yet be a reason for humans to start working on the incident. Automation can start running diagnostics that can help the response team make the right choices armed with the right information. Or, if the response to the incident is well-known, automation can even auto-remediate without any human intervention.
- Human response: If a person is needed for resolution, they should have all the relevant information at their fingertips when they begin their response. And, data should be collected from their incident response actions to help the system further learn and make the ML more productive and better able to understand future fixes.
- Probable cause analysis: When humans are responding to an incident, it helps to have ML surface key information so that responders can drive to the next best action. Based on previous incident data, ML can share data about how rare an incident is, past and related incident data, as well as change correlation from change events occuring in the system.
AIOps allows teams to proactively detect and respond to incidents in real time, helping teams achieve fewer incidents and faster resolution.
Top AIOps Tools for Incident Management
There are several AIOps tools you can use to help with incident management. These AIOps tools can help the system learn about itself more quickly and effectively in order to create smarter algorithms.
These are some of our favorite AIOps tools for incident management:
PagerDuty Process Automation
PagerDuty Process Automation works to reduce incident resolution times and minimize escalations. AIOps tools like Process Automation utilize runbook automation (RBA) to quickly and effectively diagnose and resolve incidents as they happen. PagerDuty Process Automation is a great option because of its easy setup and it integrates seamlessly with your team’s existing tools, script, and APIs. Another great feature in Process Automation is its ability to easily expand the number of people able to react to incidents, as well as their specific capabilities in responding to an incident.
Github (Puppet and Evolven)
The Github community is a great resource for finding great open source AIOps tools to integrate within your infrastructure. Puppet Automation is an open source management and deployment tool that works to automate system administration processes. Evolven is a great AIOps tool for incident detection and management. Evolven uses intelligent analytics and machine learning to detect and prioritize incidents automatically, learning overtime to predict and prevent future incidents.
PagerDuty AIOps
PagerDuty AIOps helps teams achieve fewer incidents and faster resolution. This solution reduces noise and allows teams to focus on the signals that matter, triage efficiently with better context, and automate the toil from the incident response process. It’s easy to get started, no data scientist required or lengthy implementation. It’s also valuable for any technical team, whether they’re an ITOps team, NOC, DevOps team, or distributed engineering team.
How to Get the Most Out of AIOps
AIOps tools are a great way to truly get the most out of ML and automation. These tools can integrate together within your applications and infrastructure in order to quickly learn the system and create more reliable services.
If you would like to learn more about integrating AIOps for your team, take a tour of PagerDuty AIOps or read this eBook.
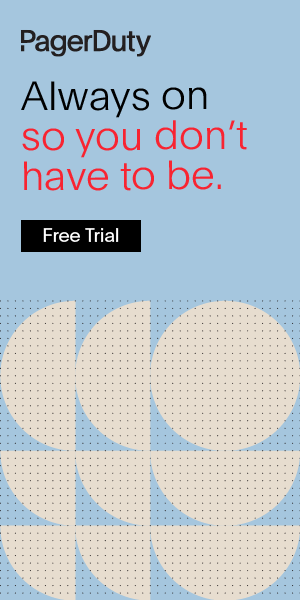
Additional
Resources
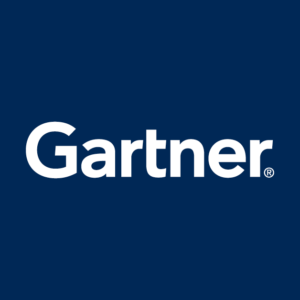
Report
Gartner® Report: 2024 Market Guide for Infrastructure Automation and Orchestration Tools
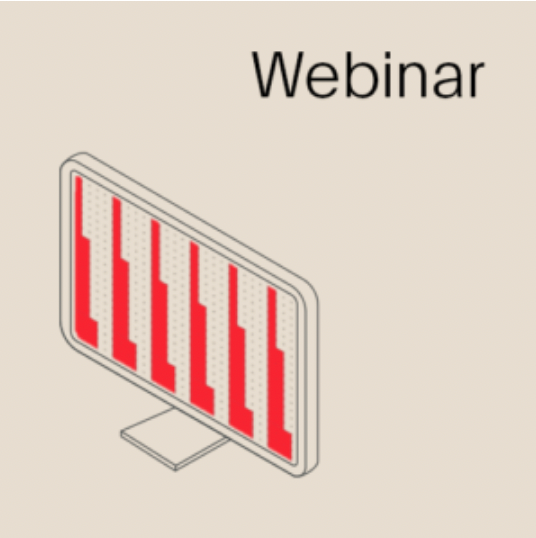
Webinar
Leveraging LLMs to Deliver Excellence in IT Operations