What is AIOps?
Today, the systems and applications within organizations generate massive volumes of data—with some organizations experiencing millions of events per day. At this scale, it is no longer viable for humans to manually parse through all that data to detect and remediate issues. The cognitive load is worsened by the fact that organizations often have dozens of tools monitoring thousands of services—any one event that emanates from these tools may be meaningless on its own. Such phenomena have created mission-critical needs for automation, machine learning, and predictive capabilities.
AIOps, or Artificial Intelligence for IT operations, is a best practice that allows organizations to improve efficiency, resolve customer impact faster, and codify incident response processes. Essentially, AIOps solutions provide similar functionality to existing event management solutions, but add capabilities required for complex, modern environments such as machine learning, flexible data collection and ingestion, end-to-end event-driven automation, and more.
How does AIOps work?
AIOps works by bringing together data from a variety of sources across an environment (including human data from response) and consolidating it into a consumable form, then automating the toil from the response process. A workflow might look like this:
Incoming data from a variety of sources is consolidated into one engine. That engine deduplicates the events, then adds an additional context to the events to normalize the information. Alerts that are not relevant (such as transient alerts) are suppressed or paused. Then, related alerts are grouped together into a single incident that’s routed to the correct team. From there, ML can provide additional triage context on the incident, and automation sequences can kick off, pulling diagnostic information or even resolving the incident entirely.
AIOps Use Cases
AIOps can be a game-changer in a variety of use cases:
- Networking Operation Center (NOC) modernization: For NOCs looking to move from eyes on glass and catch and dispatch, AIOps can be the central pane of glass to help this transition. NOCs can delegate detection, initial triage, and diagnostics to automation. NOCs can also populate incidents with the right notes and runbooks so they always feel prepared. And, with less noise, they can clearly see the signals that matter.
- Major Incident Management (MIM): AIOps can help organizations quickly detect major incidents. And, with the right context via ML, triage information and historical context gives these teams a leg up in the moments that matter most.
- Distributed service owners: Service owners have the right amount of autonomy and are able to create their own automation and noise reduction criteria to ensure that they, as the subject matter experts (SMEs), are pulled away from value-add work only when necessary.
AIOps key capabilities
Some of the key capabilities of AIOps are as follows:
- Noise reduction: Organizations should be able to reduce noise across services and eliminate interruptions caused by transient alerts or alert storms. Alerts should be grouped into relevant incidents instead of kicking off a new incident each time.
- Triage and RCA: AIOps solutions should provide users with the context needed to do their jobs faster. This includes context pulled from event data and normalized, previous historical context, and current system impact.
- Automation: Organizations should be able to create and scale automation across their technological ecosystem, reducing toil and improving efficiency. This should be able to be centrally controlled as well as available self-service for individual teams.
- Visibility: AIOps solutions should be a single pane of glass that shows you your operating posture at all times, helping you answer the all-important question, “Is my system okay?”.
Let’s delve into some of the benefits of leveraging these capabilities more specifically.
Benefits of AIOps
Overall, AIOps helps teams achieve fewer incidents and faster resolution. Here are some key benefits to keep in mind:
- Easy to get started: Ideally, AIOps shouldn’t be a long, difficult implementation. And it doesn’t need to happen overnight. Most successful implementations take a staged approach. This way, you can start seeing faster resolution and fewer incidents immediately and can reclaim that time for value-add work.
- Brings teams together: AIOps isn’t just a tool for developers. It’s equally beneficial for NOCs, ITOps teams, SREs, DevOps teams, platform engineers, and everyone. All teams have something to gain from AIOps, whether that’s less noise on the front lines or the ability to craft automation across the entire technical ecosystem.
- Continuously learning: AIOps should be a low-maintenance solution. But, that doesn’t mean once it’s set up that it’s complete. Machine learning (ML) is always operating in the background, learning about how your teams and organization resolve problems. It gets better with time.
- Shares next best actions: The best AIOps solutions don’t just give you data, they give you information and provide a next-best action. With AIOps, you know what to do next during an incident.
- Improves MTTR: With the right information at the right time, and incidents routed to the correct teams dynamically, organizations will see lower MTTR and therefore less customer impact.
- Standardizes incident response: With normalized event data, alerts, and incidents, everyone is on the same page. And, with automation to run diagnostics and ML providing triage information previously only available in old wikis and tribal knowledge, all responders can be as effective as your best responder.
- Prevents burnout: With less alert noise and alert fatigue and automation acting as an L0 responder, teams can focus on the work that matters and be interrupted less, whether they’re working on the next best feature or trying to catch up on some sleep.
AIOps challenges
With this rich potential for data science to improve your operational efficiency, your team happiness, and your organizational communication — you have to make the choice to build or buy a solution. How do you mitigate risks as well as costs in your AIOps deployment?
Building a solution is tempting — but be wary of the promise of a custom-built solution. Data science is an evolving field — what you design for your system now is almost guaranteed to be out of date by the time you deploy it. The right system requires research and experimentation that can take a while and eat up your resources in implementation and training. And if it is not being powered by enough data, it will not yield accurate insights.
Some challenges to consider when building your own AIOps tool include:
- Amount of data. For this to be successful, you need enough data to be read, scanned and analyzed. If there isn’t enough data, there won’t be a successful outcome.
- Bad quality data. Similarly, if the data isn’t good quality, machine learning can only go so far.
- Improper integrations. All legacy tools have to be modern enough that they integrate successfully with AIOps, otherwise data won’t be able to be read and used.
Consider buying a solution that fits into your current infrastructure and operational strategy — that is purpose-built based on a long history of data and experience, designed to follow best practices and adapt to your unique environment and needs. But be aware of systems that rely on extensive configuration — these often require constant management and tending.
The right AIOps solution is one that all your teams can leverage with the data you already have. And it allows you to see value immediately without a significant investment in resources up front or maintenance long term.
How to get the most out of AIOps
PagerDuty AIOps helps teams achieve fewer incidents and faster resolution with no maintenance required and no length implementations. To learn more about PagerDuty AIOps, you can watch this short on-demand webinar. Or you can see for yourself in our interactive product tour.
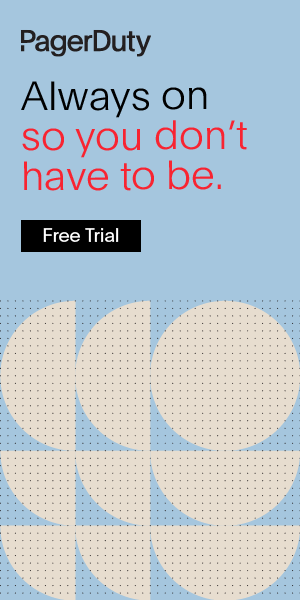
Additional
Resources
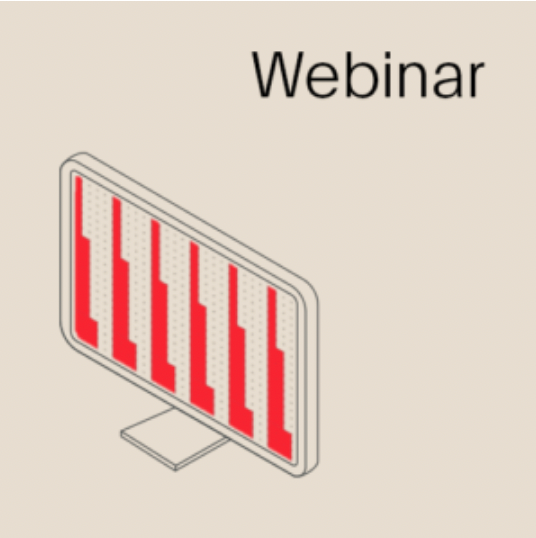
Webinar
Leveraging LLMs to Deliver Excellence in IT Operations
Whitepaper
ServiceOps Executive Playbook Modernizing IT Service Management